All its users are beginning to admit it: the answers of generative AI sometimes lack relevance and this is sometimes dangerous. To use it better, to contribute to improving its French-language versions, the Ministry of Culture has just launched an online comparator: compar:IA
As occasional users of generative artificial intelligence, we at Wazup-Intech have been able to observe several relevance flaws in the responses to our prompts that could have had significant consequences if we had not taken the trouble to check and control by conventionally soliciting search engines. From now on, everyone can request a new tool put online by the Ministry of Culture: an AI comparator. Because, the ministry justifies, “We should not rely on the answers of a single AI”…
A problem of relevance
Here are some examples from our professional activity to show you how generative AI must be used with caution and how relevant the comparison of at least two AIs, suggested by the ministry, is.
- For example, when I asked ChatGPT to write an example of an open letter to a specific minister, the proposed version began with "Dear Madam Minister". This was a double problem because this familiarity is never used in this type of letter, and – above all – the potential recipient minister at the time was a man! I learned to my cost that GPT is programmed to always provide an answer, or even invent one if it cannot find one, and never cross-check or verify its information! Unlike journalists...
- Another example, looking for innovations at a trade fair whose edition was ending (which was specified in the prompt), the examples given in responses related to previous trade fairs without this being specified.
- Another time, trying to find the statement of a major leader of a multinational, ChatGPT provided me with a specific quote which – after checking – had never been uttered by the person concerned during the event in question.
- Another time, asking for European statistical data in 2024, the answers given were not more recent than 2022, despite my insistence on having updated figures. I will have to insist that ChatGPT "admits" that it does not have access to data after 2022, which it should have done on its own.
These few examples demonstrate the frequent flaws, even the dangers of generative AI and therefore the need to both control its responses and, better still, compare them.
Compar:IA, for blind comparison
This is precisely the objective of the initiative launched by the Ministry of Culture, whose Digital Directorate has just put the Beta version of a FREE “AI Comparator” called Compar:AIIts use and purpose are simple, clear and ambitious.
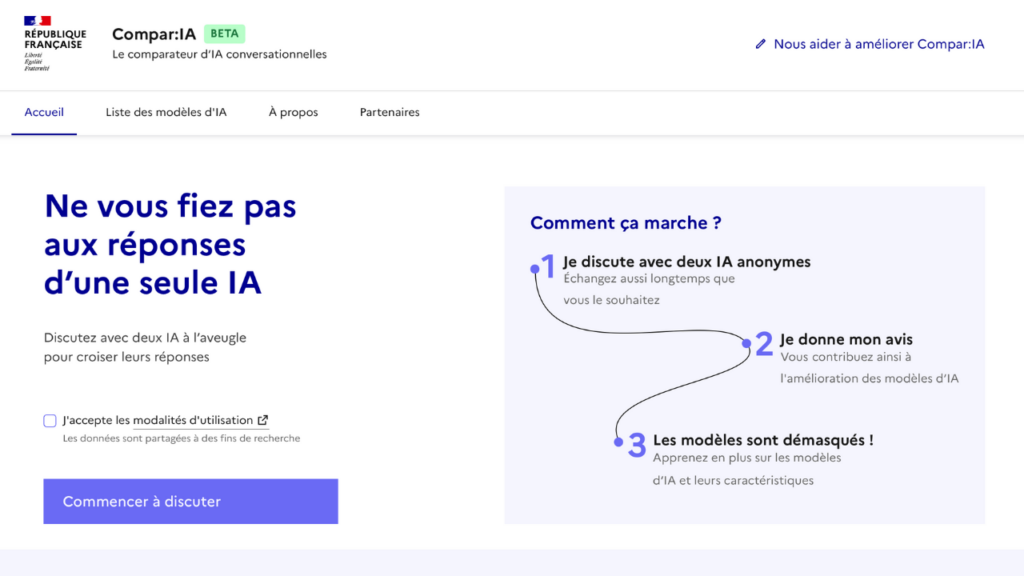
- In terms of use, it allows you to Simultaneously solicit two anonymous generative AIs on the same subject and for as long as necessary and to compare the relevance of the answers. After which, the requested AIs are revealed and their respective characteristics explained.
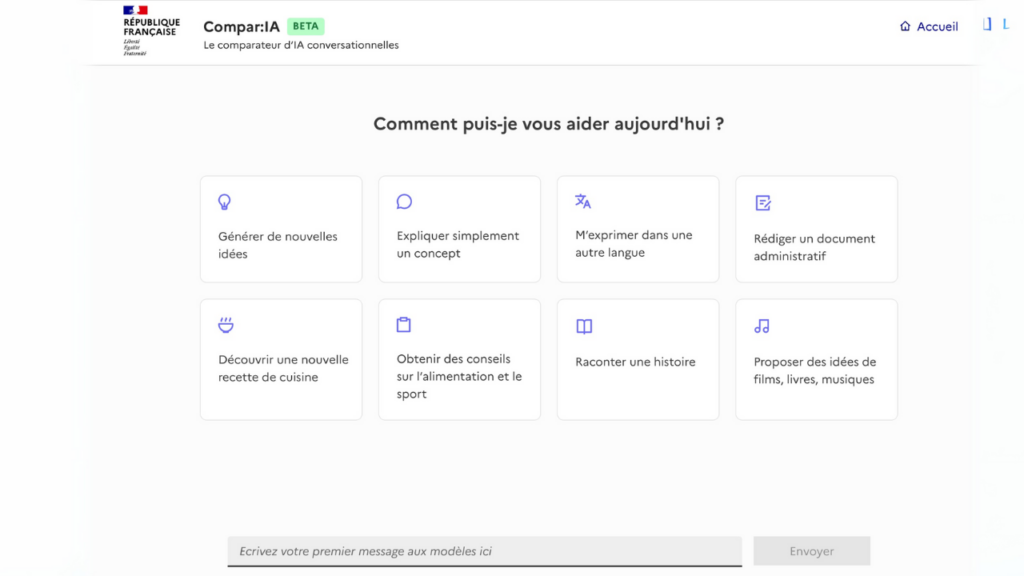
- In terms of the finality, the user is invited to indicate the best answer in order to feed a dataset, in French, concerning everyday uses. The dissemination of this data will improve the quality of language models conversational in French use.
Another claimed purpose: “ Encourage users' critical thinking by making effective a right to pluralism of models.
16 AI models to compare
The platform has referenced 16 AI models, some of which are variations of others. It includes several versions of Mixtral and Mistral Nemo, from the French Mistral, Llama (Meta), Gemma and Gemini (Google), and Chocolatine (Jpacifico), Nous (Hermès), LFM (Liquid), Phi from Microsoft and Qwen (Alibaba).
A problem of bias
Because for the Ministry of Culture, one of the reasons – it is not the only one, as we have seen – why our generative AIs are not sufficiently relevant lies in the linguistic and cultural bias. In short, the Digital Department specifies:
“Conversational AIs rely on large language models (LLMs) trained primarily on English data, which creates linguistic and cultural biases in the results they produce. These biases can result in partial or even incorrect responses. neglecting the diversity of languages and cultures, particularly French-speaking and European.”
Collect user preferences
How can we correct this bias and improve the relevance of conversational agents’ responses? By using “alignment”. Alignment is a bias reduction technique that relies on collecting user preferences to adjust AI models. so that they generate results consistent with specific values or objectives.